By Matt Day
The Seattle Times
WWR Article Summary (tl;dr) Linkedin doesn’t ask users their gender at registration, and doesn’t try to tag users by assumed gender, however, if you search for a popular female first name you will see a pattern of suggestions for a similar-sounding man’s name.
SEATTLE
Search for a female contact on LinkedIn, and you may get a curious result. The professional networking website asks if you meant to search for a similar-looking man’s name.
A search for “Stephanie Williams,” for example, brings up a prompt asking if the searcher meant to type “Stephen Williams” instead.
It’s not that there aren’t any people by that name, about 2,500 profiles included Stephanie Williams.
But similar searches of popular female first names, paired with placeholder last names, bring up LinkedIn’s suggestion to change “Andrea Jones” to “Andrew Jones,” Danielle to Daniel, Michaela to Michael and Alexa to Alex.
The pattern repeats for at least a dozen of the most common female names in the U.S.
Searches for the 100 most common male names in the U.S., on the other hand, bring up no prompts asking if users meant predominantly female names.
LinkedIn says its suggested results are generated automatically by an analysis of the tendencies of past searchers. “It’s all based on how people are using the platform,” spokeswoman Suzi Owens said.
The Mountain View, Calif., company, which Microsoft is buying in a $26.2 billion deal, doesn’t ask users their gender at registration, and doesn’t try to tag users by assumed gender or group results that way, Owens said. LinkedIn is reviewing ways to improve its predictive technology, she said.
Owens didn’t say whether LinkedIn’s members, which total about 450 million, skewed more male than female. A Pew Research survey last year didn’t find a large gap in the gender of LinkedIn users in the U.S. About 26 percent of male internet users used LinkedIn, compared with 25 percent of all female internet users, Pew said.
LinkedIn’s female-to-male name prompts come as some researchers and technologists warn that software algorithms, used to inform everything from which businesses show up in search results to policing strategies, aren’t immune from human biases.
“Histories of discrimination can live on in digital platforms,” Kate Crawford, a Microsoft researcher, wrote in the New York Times earlier this year. “And if they go unquestioned, they become part of the logic of everyday algorithmic systems.”
There’s plenty of evidence of that recently.
A Google photo application made headlines last year in mistakenly identifying black people as gorillas.
More recently, Tay, a chatbot Microsoft designed to engage in mindless banter on Twitter, was taken offline after other internet users persuaded the software to repeat racist and sexist slurs.
The impact of machine-learning algorithms isn’t limited to the digital world.
A Bloomberg analysis found that Amazon.com’s same-day delivery service, relying on data specifying the concentration of Amazon Prime members, had excluded predominantly nonwhite neighborhoods in six U.S. cities. Meanwhile, ProPublica found that software used to predict the tendencies of repeat criminal offenders was likely to falsely flag black defendants as future criminals.
People who work in machine intelligence say one of the challenges in constructing bias-free algorithms is a workforce in the field that skews heavily white and male.
“It really comes down to, are you putting the correct training data into the system,” said Kieran Snyder, chief executive of Textio, a Seattle startup that builds a tool designed to detect patterns, including evidence of bias, in job listings.
“A broader set of people (working on the software) would have figured out how to get a broader set of data in the first place.”
A few months ago, Snyder said a Textio analysis found that job postings for machine-learning roles contained language more likely to appeal to male applicants than the average technology industry job post.
“These two trains of conversation, one around inclusion in technology, the other around (artificial intelligence), have only been growing in momentum for the last couple of years,” she said.
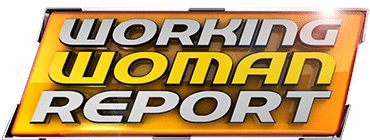